By Robert Iati, Sr. Director, Capital Markets, Dun & Bradstreet
We’ve been hearing about the importance of Big Data for what seems like decades. But often, investment banks approach data projects by placing insufficient attention on reference data, typically due to the prominent focus on ultra-fast market data and the limited amount of discretionary funds resulting from the commitment to meet overwhelming demands of compliance with capital markets regulations (such as Dodd-Frank and Volcker Rule). Here are three reasons why that’s a strategic mistake.
1. Data volumes continue to grow exponentially
After sifting through near-unmanageable volumes of data, investment banks and asset managers are now challenged to discover how to use all this data and, more importantly, how to find meaningful patterns in that data that delivers insights that others don’t have. With the focus of data placed so squarely on gaining greater insight into pricing (market data), many retain the feeling that reference data—because customer data, counterparty data, indicative product data is perceived to be less dynamic—is less important to their ability to generate trading profits. That, however, is a mistaken view.
In fact, reference data is far more complex today than at any time in our history. Every entity has so many relationships—via acquisitions, affiliations, partnerships and similar dealings—that the spider web of connections for any single entity is nearly impossible to follow. Multiply that times the number of distinct entities in the world and you quickly highlight the magnitude of the problem. The simple result is that we don’t know with whom we’re dealing when transacting trillions of dollars of securities.
This influences the counterparties with whom investment banks trade, the companies issuing the bonds they invest in, and the entire ecosystem of institutions that comprise the capital markets industry.
2. Competitive insight can yield giant returns
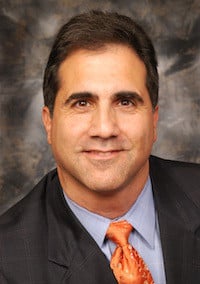
Bob Iati, D&B
In the trading business—possibly the most competitive of all—it’s all about a firm’s ability to differentiate itself using that data. We all know that the trading business has evolved (some say devolved, but I’ll refrain from that debate for now) into one where technology trumps human intelligence and quantitative process overrules fundamentals. So, in some ways, technology levels the playing field among the large firms, each of which invests hundreds of millions on trading technology annually simply to keep pace.
The well-worn fact is that alpha has become so hard to find that trading institutions go to the greatest efforts to identify an edge. However, technology too is often commoditized, making a genuine edge more difficult to gain. So if trading firms have similar technology and use the same data as do their competitors, the tide will rise but all firms will rise with it at the same rate. In trading—a zero sum game—that doesn’t satisfy. It’s relative growth that matters, leading my firm to win more frequently than my competitors. The winners are those that have the best inputs into their technology. Pairing those inputs with the most refined algorithms and trading models effectively brings out the best in that data. Finding the unique datasets—either raw or derived—is the winning formula.
3. Access to unique data enables well-informed investing
What am I talking about here? Reference data that offers insightful information about companies that form the core of our investments—equities or fixed income securities—is moving to the front of the line for investment models. It can often identify some way to identify a leading indicator for an investment position.
It’s the insight into the payment history for a private issuer of bonds that enables a trader to assess its price better than the other bidders.
It’s the ability to create new models by using analytics that combine existing (and sometimes common) data with new data—in different ways—to uncover leading indicators to a trend in a sector or that identifies a trigger for a named stock that enables that trader to benefit uniquely.
It’s about getting an early view into the glitches in the supply chain of a manufacturing company that reduces its future earnings projections and triggers a ‘sell’ signal on its stock before the rest of the market can figure it out.
The point here is that we know the data used by most trading firms is indeed quite similar. However, in spite of all other machinations adopted by trading firms to take the most advantage of their assets, all traders remain on the lookout for data that they can deploy uniquely to separate from the pack. That offers clear and measurable value.
Right now, the differentiating factor for many of these firms is in the models used by each to derive new data. That is the secret sauce they now count on to achieve alpha returns. But improving the inputs by adding genuine unique data to the equation, raises their game to its highest point.