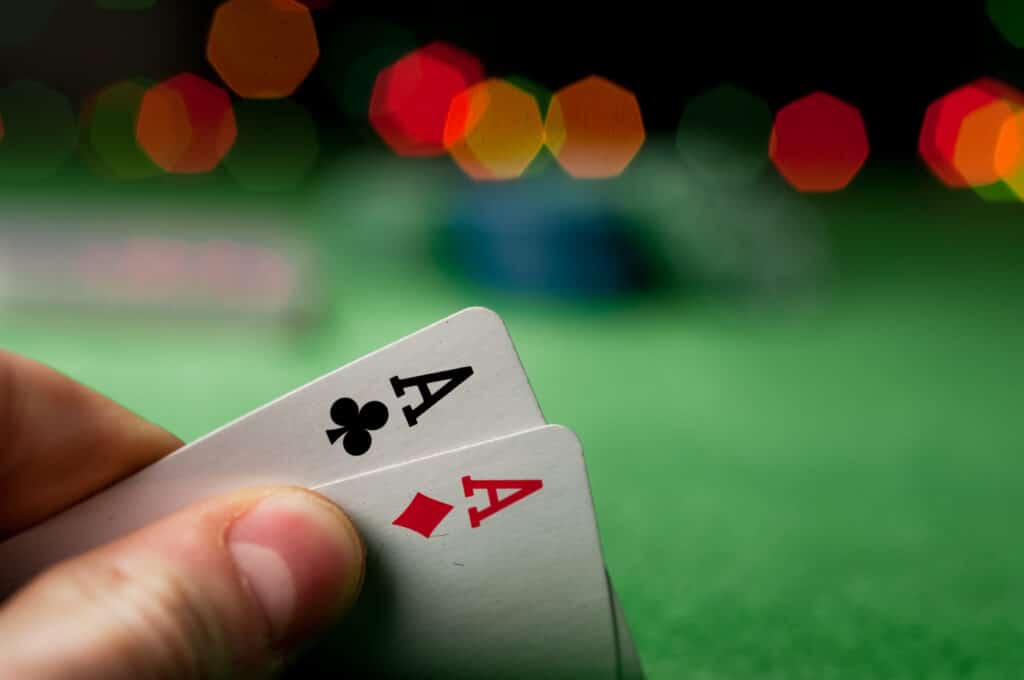
By Richard Sparrow, CFA, Director, APAC AES Coverage, and Greta Zhou, Vice President, APAC AES Product, Credit Suisse
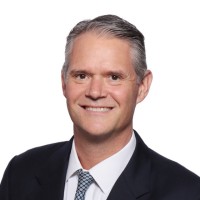
The history of computers taking on humans in games is a long one. Unfortunately for humans, it is characterized by the steady and undeniable trend of computers becoming increasingly more dominant over time.
Backgammon was the first to fall in 1979. Then went checkers 15 years later in 1994. Chess followed surprisingly closely in 1997, with IBM’s Deep Blue program taking down world champion Garry Kasparov on its second try. The ancient board game go finally succumbed in 2016.
Standing defiant in the face of this history of defeats, poker has stubbornly resisted the tide of computer domination. For example, Facebook’s Rebel, which is widely believed to be the most advanced program of its kind, showed last year that it could consistently win on average against humans, but with a thin margin and high standard deviation. In other words, in contrast to chess, go, and other board games, humans still have a solid chance of beating the best poker programs.
What makes poker so much more challenging for computers than other board games? The answer lies in the concept of information asymmetry. In each of the board games mentioned above, all players have the exact same information, or ‘perfect’ information, about the current state of play, at all points in time. In poker, there is asymmetric information: not all players share the same data. Each player knows their own state and intentions, but does not know that of the others. This prevents AI programs from calculating every possible outcome, and as a result, humans still stand a chance against the machines.
Trading in a stock market, or any other market, naturally involves asymmetric information. There are multiple players, each with their own goals and strategies, and no single participant has perfect information about the other players. If we had full transparency into the aggregate supply and demand at different price levels we could theoretically make a reasonable estimate of future price moves, but of course this is impossible.
However, we are not without clues. Just as how the five ‘community cards’ in Texas hold’em poker provides shared information to all participants, the lit order book on exchanges provides important information on supply and demand in the market at various levels.

At Credit Suisse we looked into how we could harness this order book information to help us predict future price moves and guide our posting and taking decisions. Credit Suisse recently introduced a proprietary Equilibrium Price (EP) quantitative model that uses this information to predict short-term price movements. Based on its forecasts, the model guides our trading algorithms to post inside a multi-tick spread, post at or more passively than the current near touch, or cross the spread and take liquidity.
While this approach would seem intuitive, we also needed to gauge its effectiveness and confirm how much the displayed supply and demand in the visible order book is indicative of the accurate supply and demand as evidenced by actual price moves.
Using Credit Suisse’s ‘AlgoKaizen’ framework, we compared our new EP model against other competing quantitative models at randomized child-level trials and obtained a robust and granular data set of performance metrics. The best model is now incorporated into our strategies based upon what we learned from the resulting data about when and where they performed best, thereby strengthening the core of our AES algorithmic trading suite.
So what did we learn? As expected, we found that the order book contained significant predictive value. Moreover, our EP model solidly outperformed most of the competing models across almost the entire APAC region. For example, in Hong Kong, our EP model outperformed on over 80% of Hang Seng Index stocks, while in Australia, it outperformed on over 65% of ASX200 index constituents. However, Japan was the exception. In Japan, the EP model seemed to provide less consistent price-predictive power than in the other markets, outperforming on only 45% of the Nikkei 225 stocks and less than 30% of TOPIX 100 constituents. Why was this?
In Japan, the EP model competitor was our Adaptive Trade Velocity (ATV) model which served as a control for the AlgoKaizan trials. The ATV model makes less use of the order book but more historical trade velocity and real-time trading events to make decisions. In practice, it will usually adhere more tightly to its target trading trajectory, relying less on what the order book may be showing at the time.
Figure 1, below, helps shed some light on what we discovered from this comparison. It shows the results of our ‘AlgoKaizen’ trials, breaking down our EP model performance by different microstructure and the trading characteristics of individual stocks. A green or purple ‘peak’ in the diagram suggests that our EP model ‘Won’, i.e. it offers superior price-prediction information and indicates that the order book does indeed contain meaningful insight into market supply and demand. In other cases, the EP models offer no advantage, or in the case of a blue or yellow peak it ‘lost’, or underperformed our ATV model. See Figure 1.
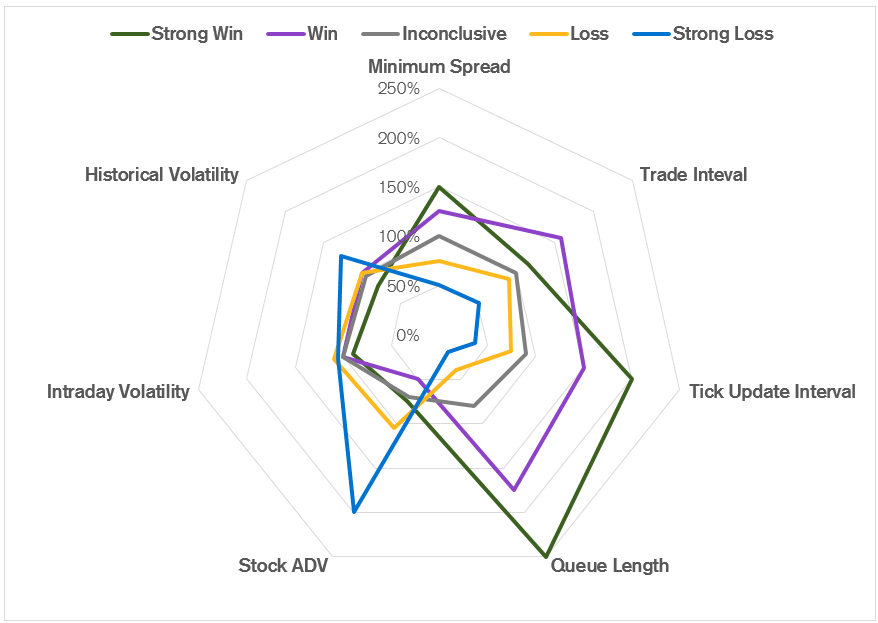
We observe strong wins for our EP model for stocks with longer queues, wider spreads, and more time between trades. Conversely the ATV model wins for stocks with high intraday and historical volatility and high trading volumes. In other words, the displayed order book seems to provide more useful information to participants in Japan for stocks that are more stable with infrequent trades and wider spreads than for stocks with higher volatility, fast turnover, and narrower spreads.
Looking at the breakdown of which model was most affected by Japan index membership, consider Figure 2.
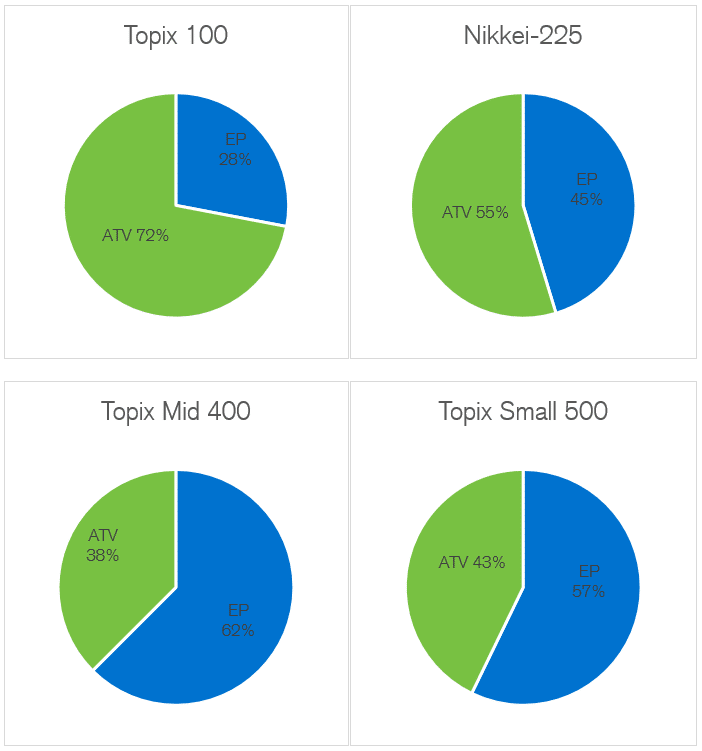
The pie charts show the percentage of stocks for which each model proved to have superior price-prediction ability for each index. As mentioned, for most large-cap stocks, particularly in the TOPIX 100, the order book did not seem to contain useful information. This is perhaps to be expected given the results from Figure 1; the TOPIX100 names have a narrow 4bp average tick size and are highly liquid. However, as we scale down to small and mid-cap names, we see more representative supply and demand information in the order book, and correspondingly a higher ‘winning’ percentage for our EP model.
Why is there more information in the displayed order book for these small and mid-cap stocks against others? The answer may lie in the tradeoffs involved when one decides whether to 1) post in the order book, or 2) not post and instead wait to take liquidity. Some stocks may trade in ways that incentivize participants to share information about their intentions via posting in the order book, and other stocks do not.
Let’s consider a small-cap stock with infrequent trading and a wide spread. It intuitively makes sense in this case for a trader to post and capture potential near-touch fills instead of immediately paying the spread, despite the information leakage that results from that decision. Therefore participants trading these names will be more likely to post, thus imparting more information to the order book for these names. The aggregate result is that the order book should contain a reasonable estimation of true supply and demand in that stock. As individual stock characteristics move towards large caps with more liquidity and frequent price movements, the advantages of posting and being present in the order book diminish relative to the costs associated with information leakage. Consequently the lit order book may not provide as much information on the true market supply and demand for that stock.
So as stock and market microstructure characteristics vary across the APAC trading universe, and shift over time, participants can be incentivized to disclose more or less of their intentions to other participants in order to achieve best execution. The challenge for us as traders is not just to read the information available to us, but also to know the extent to which it is representative of the market and form a basis for decision making. In other words, we have to be not only good at playing both chess and poker but also know when to apply the lessons from each.
The combination of our new EP model and AlgoKaizen methodology improves our platform’s ability to successfully identify the game being played and to also apply the winning strategy most appropriate for that particular game.
Does the Stock Market Tip Its Hand? What the Order Book Tells Us — and What It Doesn’t first appeared in the Q3 issue of GlobalTrading.